・A large dataset describing the bacterial growth in a broad environmental gradient of largely varied combinations was experimentally acquired under well-controlled laboratory conditions.
・By applying machine learning with artificial intelligence to this big data to estimate which nutrient determinative affected E. coli proliferation, we found that the decision-making nutrients in the lag, exponential, and stationary phases differed.
・Differentiation in the elements determining various growth phases strongly implied risk diversification in fate decisions as a survival strategy.
Abstract
Microorganisms growing in their habitat constitute a complex system. How the individual constituents of the environment contribute to microbial growth remains largely unknown. The present study focused on the contribution of environmental constituents to population dynamics via a high-throughput assay and data-driven analysis of a wild-type Escherichia coli strain. A large dataset constituting a total of 12,828 bacterial growth curves with 966 medium combinations, which were composed of 44 pure chemical compounds, was acquired. Machine learning analysis of the big data relating the growth parameters to the medium combinations revealed that the decision-making components for bacterial growth were distinct among various growth phases, e.g., glucose, sulfate, and serine for maximum growth, growth rate, and growth delay, respectively. Specifically, “starting to proliferate quickly”, “proliferating rapidly”, and “proliferating abundantly” are determined separately by nitrogen sources, sulfur, and carbon sources, respectively. Further analyses and simulations indicated that branched-chain amino acids functioned as global coordinators for population dynamics, as well as a survival strategy of risk diversification to prevent the bacterial population from undergoing extinction.
Benefit
In this study, by experimentally mimicking complex environmental factor changes in nature, we discovered a more universal cellular proliferation model and revealed a rational survival strategy that bacteria possess to proliferate under diverse environments—risk diversification. This is expected to provide a foundation for future cellular proliferation research.
Market Application
In this study, by experimentally mimicking complex environmental factor changes in nature, we discovered a more universal cellular proliferation model and revealed a rational survival strategy that bacteria possess to proliferate under diverse environments—risk diversification. This is expected to provide a foundation for future cellular proliferation research.
Publications
https://elifesciences.org/articles/76846
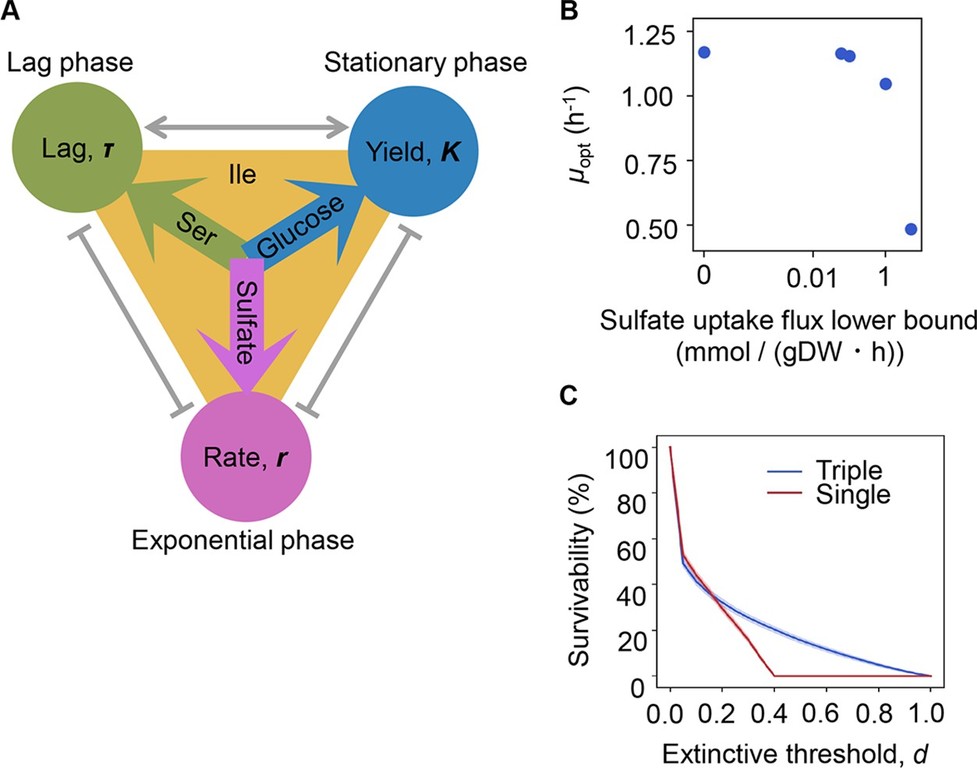
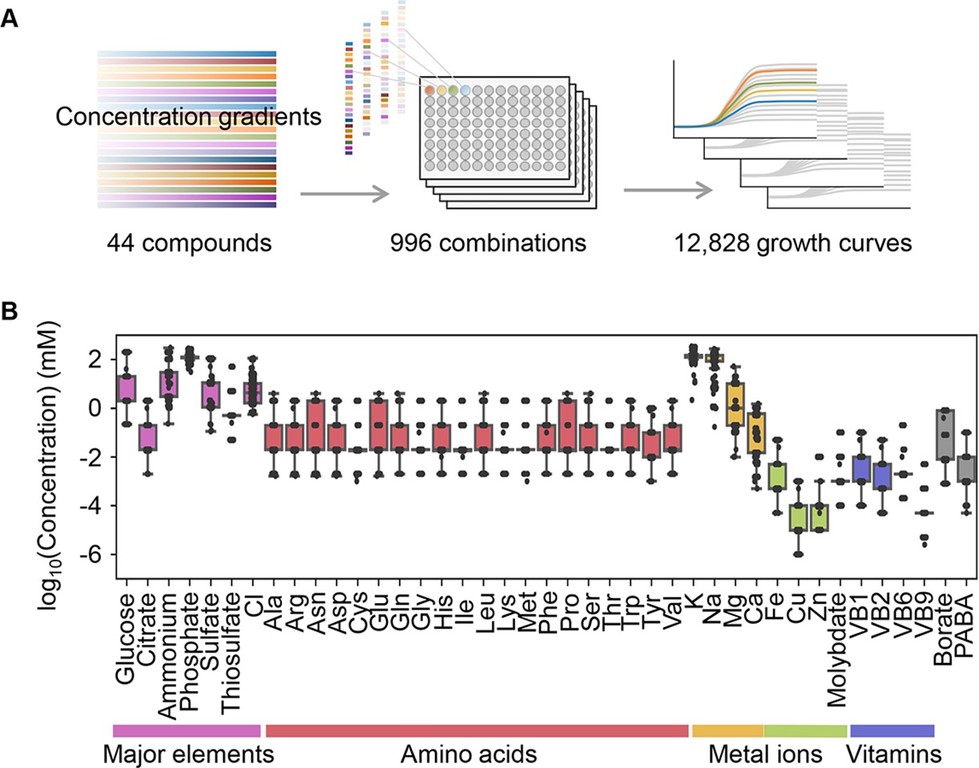
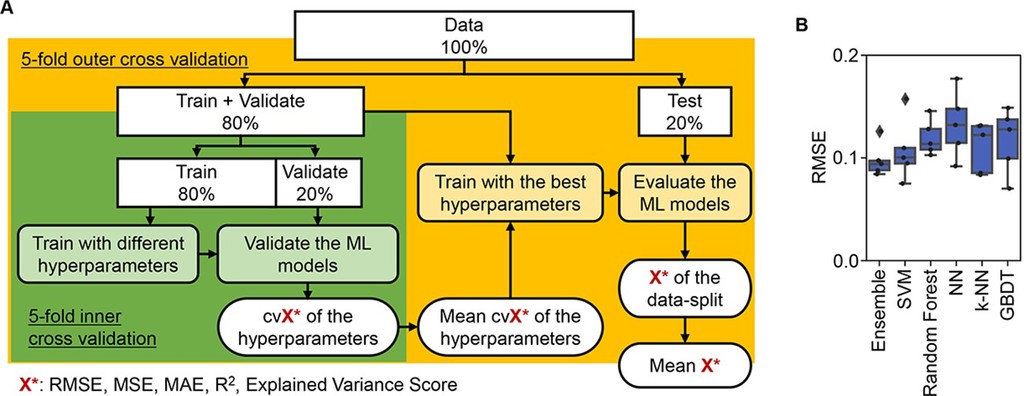
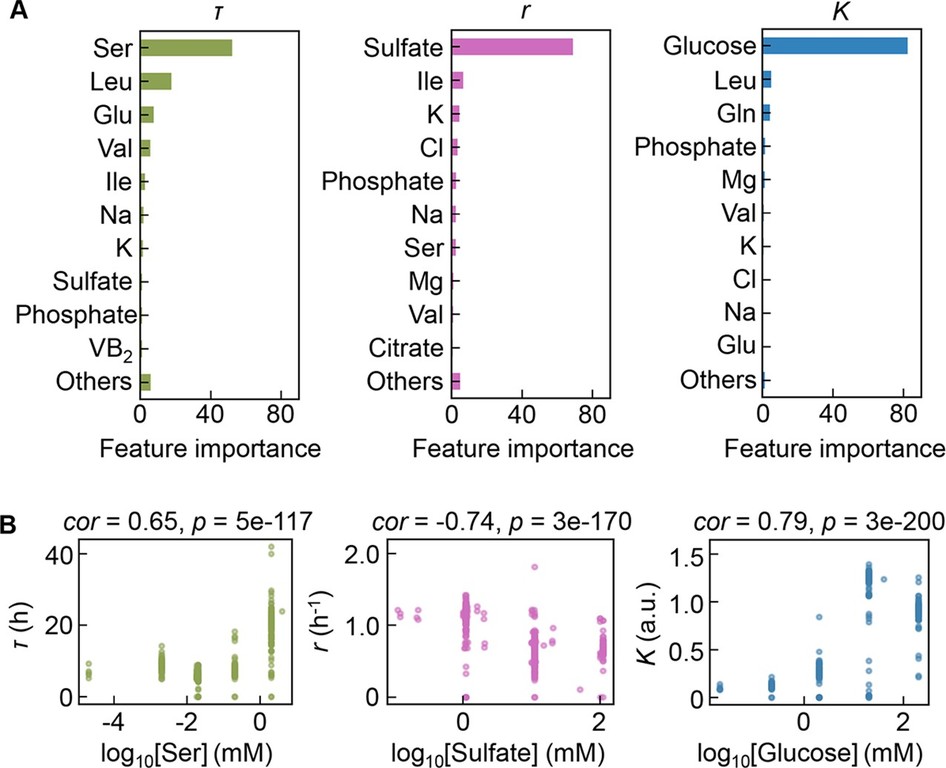